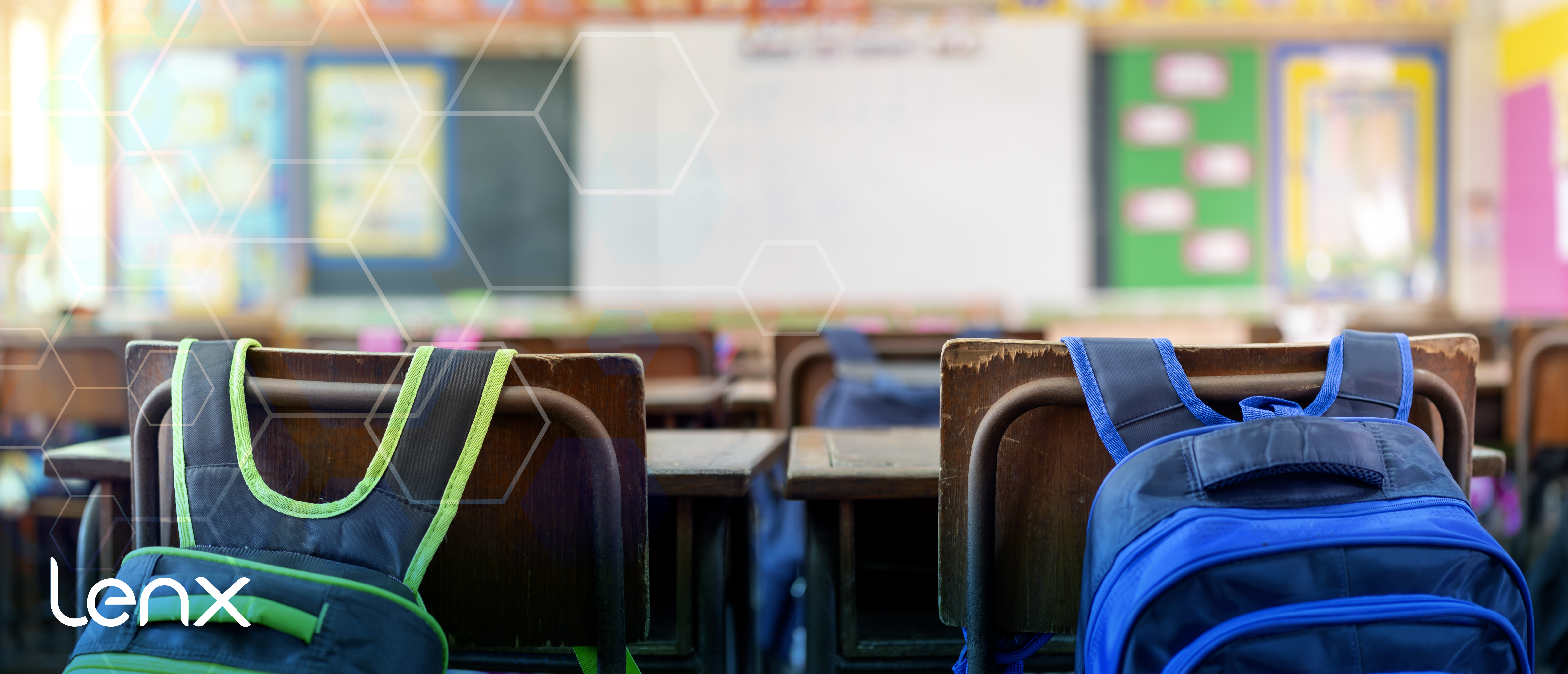
How False Alarms Can Erode Trust In AI Security And Active Shooter Detection Systems
AI security systems, including active shooter detection, are becoming increasingly prevalent. They promise to enhance safety and response times in critical situations.
However, these systems are not infallible. False alarms can occur, causing unnecessary panic and resource wastage.
These false alarms can erode trust in AI security technologies. They can also lead to complacency, reducing the effectiveness of real alerts.
In this article, we delve into the impact of false alarms on AI security and active shooter detection systems. We'll explore how to minimize these incidents and maintain trust in these crucial technologies.
Understanding False Alarms in AI Security
False alarms in AI security refer to instances where the system incorrectly identifies a threat. This could be an AI gun detection system misidentifying an object as a firearm.
These false positives can occur due to various factors. It could be due to algorithmic errors, poor quality training data, or environmental factors affecting the system's sensors.
Understanding the causes of these false alarms is crucial. It helps in refining the AI systems and reducing the occurrence of such incidents.
The Impact of False Alarms on Trust and Response
False alarms can have a significant impact on trust in AI security systems. When a system frequently generates false positives, users may start to doubt its reliability.
This erosion of trust can lead to complacency. Users may start to ignore alerts, assuming they are likely to be false. This could have serious consequences in the event of a real threat.
False alarms also strain resources. They can lead to unnecessary deployment of emergency response teams, causing fatigue and resource wastage.
In the context of active shooter detection, false alarms can cause unnecessary panic and fear. This can disrupt normal operations and create a stressful environment.
Case Studies: When AI Gets It Wrong
In one instance, an AI security app misinterpreted a drill as a real threat. This led to a lockdown and widespread panic before the error was identified.
In another case, an AI gun detection system repeatedly triggered false alarms. This caused unnecessary stress and disruption, leading to a loss of faith in the system.
These cases highlight the potential consequences of false alarms in AI security and the importance of accuracy in threat detection.
Balancing Sensitivity and Specificity in AI Systems
AI security systems must strike a balance between sensitivity and specificity. High sensitivity means the system can detect real threats, but it may also trigger false alarms.
On the other hand, high specificity reduces false alarms. However, it may also miss real threats. This balance is crucial for maintaining trust in AI security systems.
Achieving this balance requires continuous learning and improvement in AI algorithms. This ensures that the system can accurately distinguish between real threats and benign activities.
Minimizing False Alarms: Best Practices for AI Security
Minimizing false alarms in AI security involves several best practices. These include the use of robust training data and continuous system monitoring.
AI security systems should be trained with diverse and representative data. This improves their ability to accurately detect real threats.
Here are some best practices for minimizing false alarms:
- Use diverse and representative training data
- Implement continuous system monitoring and maintenance
- Incorporate human oversight for verifying AI-generated alerts
- Test and validate the system before full-scale implementation
- Train users to correctly interpret and respond to alerts
These practices can help reduce false alarms and build trust in AI security systems.
The Future of AI in Reducing False Alarms
The future of AI in reducing false alarms looks promising. Advancements in AI technology are expected to lower false alarm rates.
AI systems are continually learning and improving. This ongoing refinement, coupled with community feedback, can enhance the accuracy of threat detection.
In the end, the goal is to create AI security systems that are reliable, precise, and trusted by users.
Conclusion: Building Trust Through Reliable AI Security
Building trust in AI security systems is crucial. This trust can be achieved through the development of reliable and accurate systems.
In conclusion, while false alarms pose a challenge, they also drive the need for continuous improvement in AI security.